Data Science Benefits in Trading: Unleashing the Power of Predictive Analytic
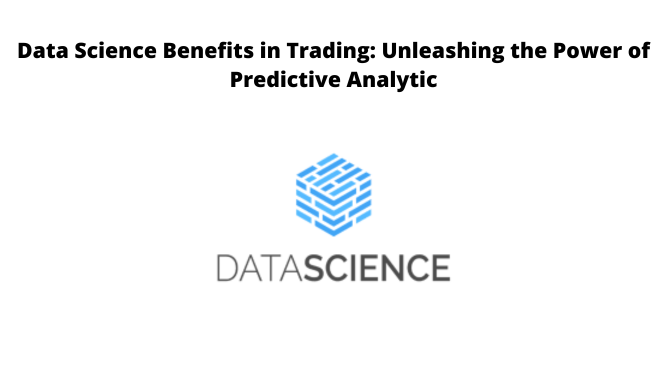
Introduction
Data science has permeated virtually every industry, and trading is no exception. In financial markets, where timely decisions can translate into substantial gains or losses, data-driven insights have become a game-changer.
In this blog post, we will delve into the benefits of data science in trading, highlighting how predictive analytics, machine learning, and advanced data analysis techniques are reshaping the landscape of financial markets. Become an early leader in the Data Science domain by joining the intense Data Science Training in Hyderabad course by Kelly Technologies.
The Role of Data Science in Trading
Data science, a multidisciplinary field that combines expertise in data analysis, statistics, machine learning, and domain knowledge, plays a pivotal role in trading for several reasons:
-
Data Abundance: Financial markets generate vast amounts of data, from historical price charts to news feeds and social media sentiment. Data science techniques help sift through this wealth of information to identify valuable insights.
-
Prediction and Forecasting: Predictive analytics and machine learning models can identify market trends, price movements, and trading opportunities. These models provide traders with actionable insights for informed decision-making.
-
Risk Management: Data science tools are essential for assessing and managing risks in trading. By analyzing historical data and market conditions, traders can implement strategies to mitigate losses.
-
High-Frequency Trading: In high-frequency trading, where decisions must be made in microseconds, data science algorithms can execute complex trading strategies at lightning speed, capitalizing on fleeting market opportunities.
-
Algorithmic Trading: Algorithms powered by data science can execute predefined trading strategies automatically, removing human emotions from the equation and ensuring consistent trading performance.
Benefits of Data Science in Trading
-
Predictive Analytics:
Predictive analytics, powered by machine learning models, can forecast price movements, identify trading signals, and generate buy/sell recommendations. Traders can use these insights to make data-driven decisions.
-
Quantitative Analysis:
Data science enables quantitative analysis of historical data, uncovering patterns and correlations that may not be evident to human traders. This analysis provides a foundation for developing trading strategies.
-
Risk Assessment:
Risk management is critical in trading, and data science models can assess portfolio risk and identify potential vulnerabilities. Traders can use these insights to adjust their positions and protect their investments.
-
Automated Trading:
Data science powers automated trading systems that execute trades based on predefined criteria. This reduces human error, ensures consistency, and allows for 24/7 trading.
-
Sentiment Analysis:
Sentiment analysis of news and social media data can provide insights into market sentiment and public perception. Traders can gauge market sentiment and make informed decisions based on public sentiment.
-
Market Anomaly Detection:
Data science can detect market anomalies, irregularities, and price deviations from the norm. This helps traders identify arbitrage opportunities and execute profitable trades.
-
Trade Execution Optimization:
Data science models optimize trade execution by minimizing slippage and transaction costs. This leads to improved trading performance and higher profitability.
-
Backtesting and Strategy Development:
Data science tools facilitate the backtesting of trading strategies against historical data, allowing traders to refine their strategies and assess their performance before deploying them in live markets.
-
Portfolio Management:
Data-driven portfolio management tools help traders diversify their investments, manage asset allocation, and optimize their portfolios for risk and return.
-
Real-time Market Monitoring:
Data science solutions provide real-time monitoring of market conditions and trading activity, enabling traders to respond quickly to changing market dynamics.
Conclusion
Data science has redefined trading by providing traders with data-driven insights, predictive analytics, and risk management tools. The benefits of data science in trading are evident in improved decision-making, risk mitigation, and the ability to capitalize on market opportunities. As financial markets continue to evolve and become increasingly data-centric, the role of data science in trading will only become more prominent. Traders who leverage data science capabilities will have a competitive edge in navigating the complexities of modern financial markets and achieving their investment objectives